Google plans to release new ‘open’ AI models for drug discovery
Google's TxGemma aims to revolutionize drug discovery using AI models.
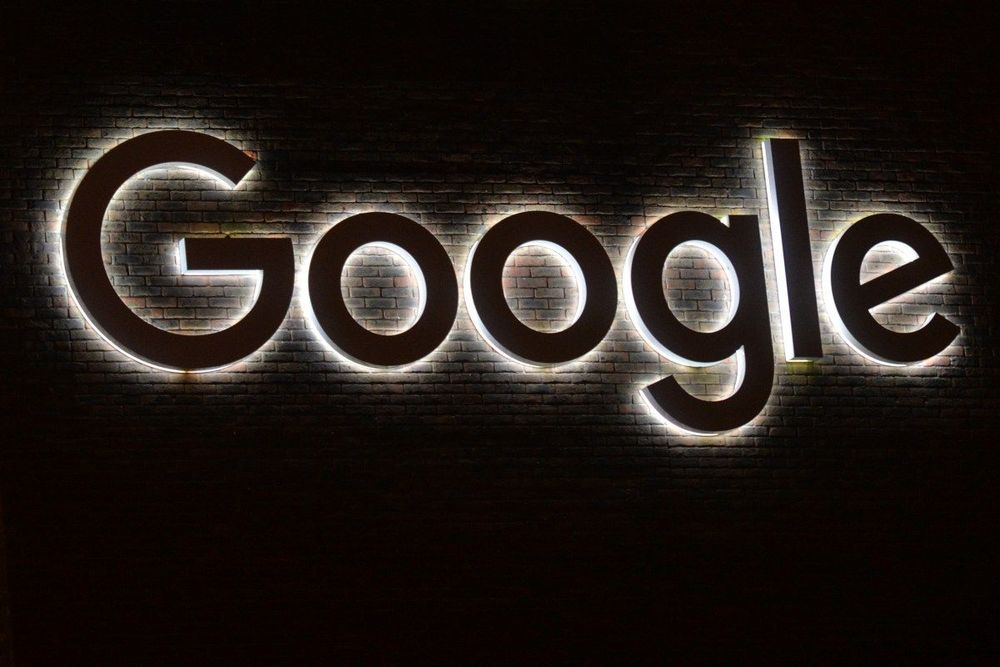
On Tuesday during a health-centered event held in New York, Google made a significant announcement regarding its development of a range of 'open' AI models tailored to aid in the field of drug discovery, collectively named TxGemma. These AI models are poised to be made available through Google's Health AI Developer Foundations program before the month's end. Notably, these revolutionary models possess the capability to process and understand not only plain 'regular text' but also the complex structures of various 'therapeutic entities,' such as chemicals, molecules, and proteins, as outlined by the company's official statement. Karen DeSalvo, Google's chief health officer, shared insights in a blog for TechCrunch, emphasizing the lengthy and costly process essential for moving therapeutic drugs from the conceptual phase to attaining approved clinical usage, hence the importance of collaborating with the broader research community to enhance efficiency at various stages of drug development. TxGemma allows researchers to pose queries regarding the prediction of critical properties of prospective new therapies, aiding in assessing their safety and efficacy.
However, clarity regarding the specifics of the model's license remains ambiguous, including whether it permits commercial use, customization, or even fine-tuning. While TechCrunch reached out to Google for further clarification on this matter, responses from Google remain pending. This announcement also surfaces amid a backdrop of both optimism and challenges within the AI-driven drug discovery industry. While many companies, including the Google spin-out Isomorphic Labs, have made laudable strides, promising swift advancements in the initial phases of research and development (R&D) through AI, the journey has not been devoid of setbacks. A notable example is the instance where companies like Exscientia and BenevolentAI faced high-profile clinical trial disappointments despite employing AI-based approaches, a testament to the fact that AI, while transformative, does not instantly provide magical solutions in laboratory settings.
Furthermore, the precision of leading AI systems in drug discovery, such as Google's DeepMind's AlphaFold 3, varies considerably, raising valid questions on reliability across different scenarios. Nonetheless, a significant show of confidence in this burgeoning technology comes from notable pharmaceutical entities and prospective investors who express optimism about its latent potential. For instance, in January, Isomorphic announced its collaborative endeavors with pharmaceutical behemoths like Eli Lilly and Novartis, conveying expectations to initiate testing on drugs designed with AI inputs this year. This optimism is substantiated by industry-related estimates, indicating the robust presence of over 460 AI-driven startups engaged in drug discovery efforts, a sector into which investors have cumulatively funneled $60 billion, underscoring the substantial financial interest that it commands.
The race towards revolutionizing drug discovery using AI models marks a trending intersection between technology and health, resonating with tech companies looking to harness generative AI for societal good. The potential for transformative impacts in medicine reflects a contemporary drive toward scientific innovation, aligning with broader trends seen in technology's capacity to offer paradigm shifts in existing processes. However, underlining such prospects is the ever-present need for thorough research, collaboration, and continuous refinement, crucial to ensuring reliable, responsible, and breakthrough developments tailored to complement and enhance existing drug discovery frameworks efficiently.
Achieving such milestones can further facilitate an enriched dialogue on maximizing the potential benefits of AI innovations within healthcare while maintaining ethical considerations to ensure safe, effective, and sustainable outcomes across diverse contexts. Introspective learning from trials and challenges faced can steer advancements positively, laying down a trodden path for emerging capabilities to claim materialized benefits for the healthcare sector and beyond in the near future.
Sources: TechCrunch, Google Blog