Meta’s vanilla Maverick AI model ranks below rivals on a popular chat benchmark
Meta's vanilla AI model is outperformed by rivals in a benchmark test.
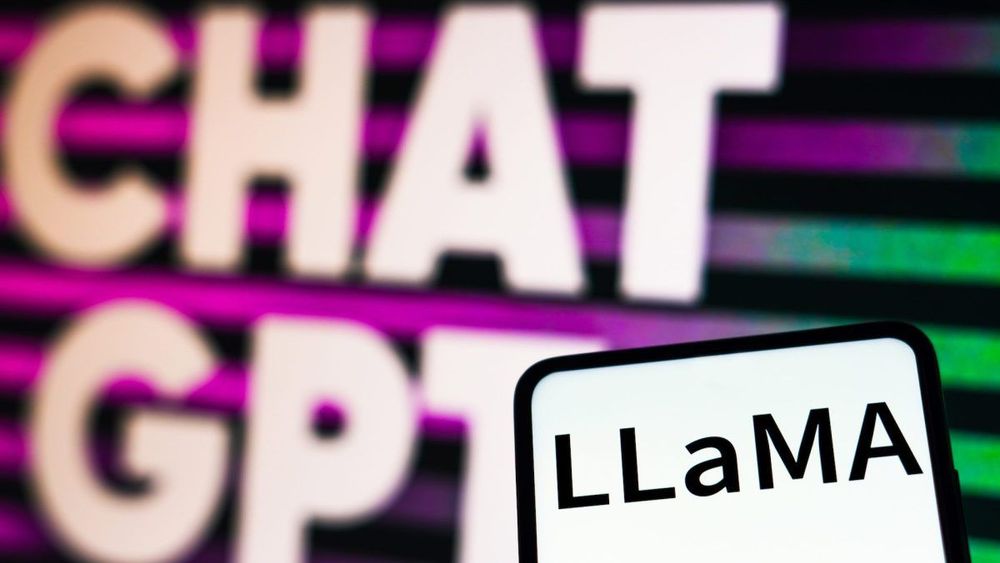
Meta faced an issue when it was revealed that the company used an unreleased, experimental version of the Llama 4 Maverick AI model on LM Arena, a popular crowdsourced benchmark. This version, known as the ‘Llama-4-Maverick-03-26-Experimental,’ was specifically optimized for conversational capabilities, making it perform exceptionally well in this benchmark. However, this led to accusations that the company may have manipulated results to present the model in a more favorable light, prompting LM Arena to update its policies.
Once this experimental version was disregarded, Meta submitted the unmodified version of Llama 4, labeled ‘Llama-4-Maverick-17B-128E-Instruct,’ for evaluation. When assessed, it ranked surprisingly low—32nd place—on the leaderboard, positioned behind elder products from competitors like OpenAI’s GPT-4o, Anthropic’s Claude 3.5 Sonnet, and Google’s Gemini 1.5 Pro. This outcome highlighted the stark performance contrast between the customized and the vanilla models.
The benchmark in question, LM Arena, operates by having human raters directly compare AI outputs and determine their preferences, which allows for subjective assessments. Its reliability as a definitive measure of AI capabilities has been critiqued, as the benchmarking environment does not replicate all real-world scenarios effectively. Models adapted specifically for such benchmarks can often struggle when evaluated in broader competitive environments.
Despite this, Meta remains unfazed. A company spokesperson conveyed to TechCrunch that experimentation is routine and includes developing tailor-made variants. Meta remains optimistic about its future, especially regarding the adaptation possibilities and customizations that developers could explore with its open-source versions of the models. The company expressed enthusiasm in anticipating feedback from users who will deploy these models in their respective applications.
The incident emphasizes the inherent challenges of using benchmarks as precise indicators of AI efficacy across various tasks. While benchmarks like LM Arena offer a glimpse into potential performance, they can sometimes provide a skewed image if models are optimized solely for these specific evaluations, thus calling for broader testing standards in the AI field.
Sources: TechCrunch, LM Arena, X