OpenAI research lead Noam Brown thinks certain AI ‘reasoning’ models could’ve arrived decades ago
Noam Brown argues AI reasoning models could've emerged 20 years earlier.
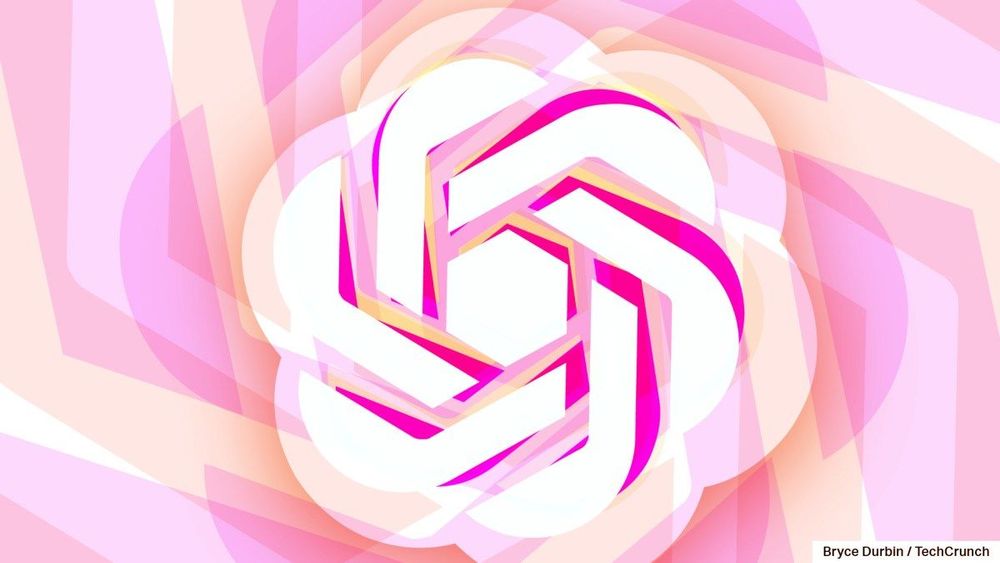
OpenAI research lead Noam Brown suggests that AI reasoning models could have been realized two decades earlier if researchers had had the foresight to pursue the right combination of approaches and algorithms. During a panel discussion at Nvidia’s GTC conference in San Jose, Brown elaborated on how academic neglect of this direction delayed advancements. He hypothesized that incorporating human-like deliberation into AI, where significant time is spent thinking before acting, could have offered substantial benefits.
Brown’s insights stem from his pioneering work at Carnegie Melon University on game-playing AI, notable for creating Pluribus, the first AI to defeat elite human poker professionals. This breakthrough marked a departure from brute-force methods towards models that 'reasoned' through complex scenarios. At OpenAI, Brown contributed to developing the model o1, which employs the technique of test-time inference to simulate a reasoning process before generating responses.
Academics, according to Brown, face unprecedented challenges in competing with AI labs like OpenAI due to resource constraints on computation-heavy experiments. He advises academic institutions to focus on less resource-intensive explorations, such as model architecture design, where they can still significantly contribute. He sees potential for fruitful collaboration between AI frontier labs and academia by drawing on academic research for concepts that can be scaled effectively.
The discussion also touched on current issues, including policy decisions such as the Trump administration’s budgetary cuts to science funding, criticized by AI figures like Nobel Laureate Geoffrey Hinton for jeopardizing both domestic and international AI research. Brown identified AI benchmarking as a critical area for academia, where impactful advances could occur without extensive computational power. Presently, benchmarks are primarily criticized for not aligning accurately with the practical capabilities of AI models, exacerbating confusion and misinterpretation of AI progress.
Brown’s reflections call for broader acknowledgment of academic contributions within larger AI research ecosystems, emphasizing the need for computational and funding support. He argues for strategic investments in AI advancements to rectify past oversights and meet future technological demands.
Sources: OpenAI, Nvidia GTC Conference, Carnegie Melon University, White House