The strange world of AI hallucinations
AI hallucinations risk incorrect, believable outputs affecting critical decisions.
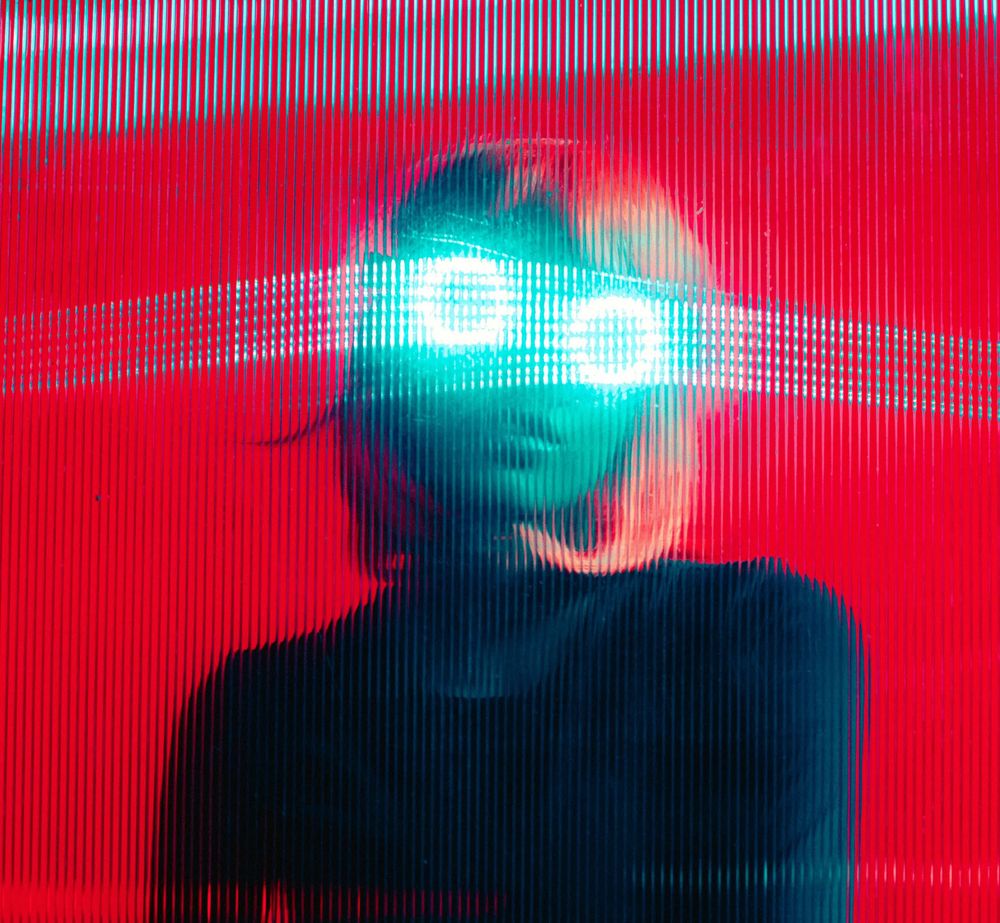
The phenomenon of AI hallucinations involves AI systems generating outputs that, while sounding truthful and believable, are incorrect or misleading. These occurrences have been documented across various AI applications, including large language models like ChatGPT, which may create nonexistent references, and image recognition software, which can mistakenly identify objects. The stakes of these errors are highest in situations where AI is employed in critical decision-making roles, such as in courts or healthcare systems, where hallucinations can lead to potentially life-altering consequences.
Contributors Anna Choi and Katelyn Xiaoying Mei, information science PhD students, note that hallucinations in AI systems can emerge when these systems use biased or incomplete training data, or when they attempt to fill gaps based on similar contexts from their training data. For example, researchers found that an AI could misidentify a blueberry muffin as a chihuahua, showing how easily errors can be made in image recognition. The issue, according to Choi and Mei, extends beyond simple misinformation into legal and ethical dilemmas where incorrect AI outputs can influence judicial decisions or wrongly assess patient insurance eligibility.
Various examples illustrate the prevalence of AI hallucinations, such as a case in 2023 involving a New York attorney who submitted a legal brief assisted by ChatGPT, which referenced a fabricated case. This incident highlights potential ramifications in legal proceedings if hallucinations go undetected. Regarding image recognition, a common example involves a system misinterpreting a scene—for instance, describing a woman talking on a phone while guessing she is sitting when no such detail exists, underlining the need for accuracy in visual data interpretations.
The causes of these hallucinations are linked to how AI systems are developed. Choi and Mei describe the process of using enormous datasets to pattern train AI, which inadvertently leads to hallucinations when faced with unfamiliar situations. Machine learning research shows that such systems can make false equivalences due to incomplete training, necessitating a critical examination of the data fed into AI to prevent misconstructions. As the deployment of AI continues to broaden, addressing these hallucinatory outputs becomes more crucial, especially where precision is paramount.
To mitigate risks associated with AI hallucinations, the authors suggest implementing stringent guidelines for AI responses and using high-quality training data, yet they emphasize continuous vigilance by users to outsmart these flaws. Double-checking AI outputs against reliable sources, especially in environments like healthcare and law enforcement, is critical. The key recommendation remains: verify and critically assess AI-generated information to intelligently integrate AI without compromising the accuracy and reliability crucial for decision-making.
Sources: The Conversation, TechSpot, Machine Learning Research Papers, Court Case Records, AI Development Studies