Why RAG won’t solve generative AI’s hallucination problem
RAG can't fully solve AI hallucination issues, has limitations and is not a perfect fix.
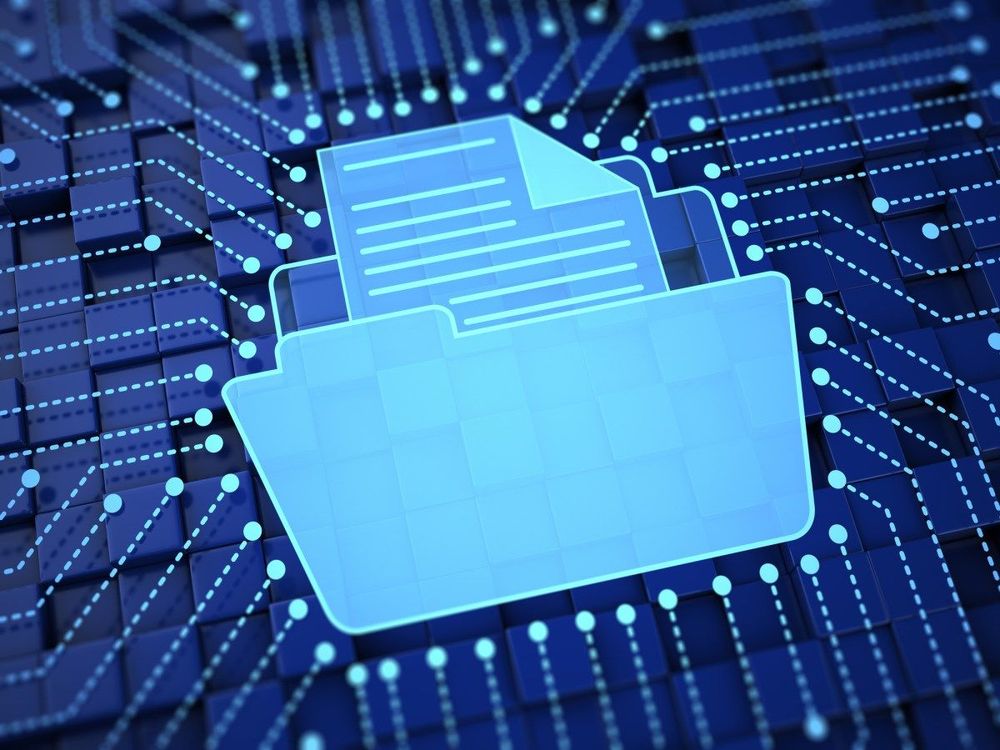
The concept of Retrieval Augmented Generation (RAG) has been heralded by some in the generative AI industry as a potential solution to the problem of AI hallucinations — false or inaccurate information generated by AI. By using RAG, the idea is that AI systems can reference existing documents to ensure the information they generate is based on factual data. However, despite these promises, RAG faces significant limitations. Specifically, it can struggle with complex reasoning tasks where keyword searches are insufficient to retrieve relevant documents, and it may inadvertently ignore useful information or get distracted by irrelevant content in the documents it does retrieve.
Moreover, the implementation of RAG is not without its costs. The technology requires significant computational resources to store and process the retrieved documents before generating a response, leading to concerns about the environmental impact and financial costs associated with its use. Despite these challenges, research into improving RAG continues, with efforts focusing on better document retrieval methods and more efficient use of retrieved information by AI models.
In conclusion, while RAG offers a novel approach to mitigating some of the inaccuracies and falsehoods generated by AI models, it is not a panacea. It performs best in scenarios with clear, knowledge-intensive questions but falls short in more abstract or complex reasoning tasks. Furthermore, the additional computational demands and the potential for models to still ignore retrieved data highlight that RAG is an evolving tool rather than a complete solution to generative AI's hallucination problem. Businesses and researchers should remain cautious of claims that RAG can entirely eliminate AI inaccuracies.